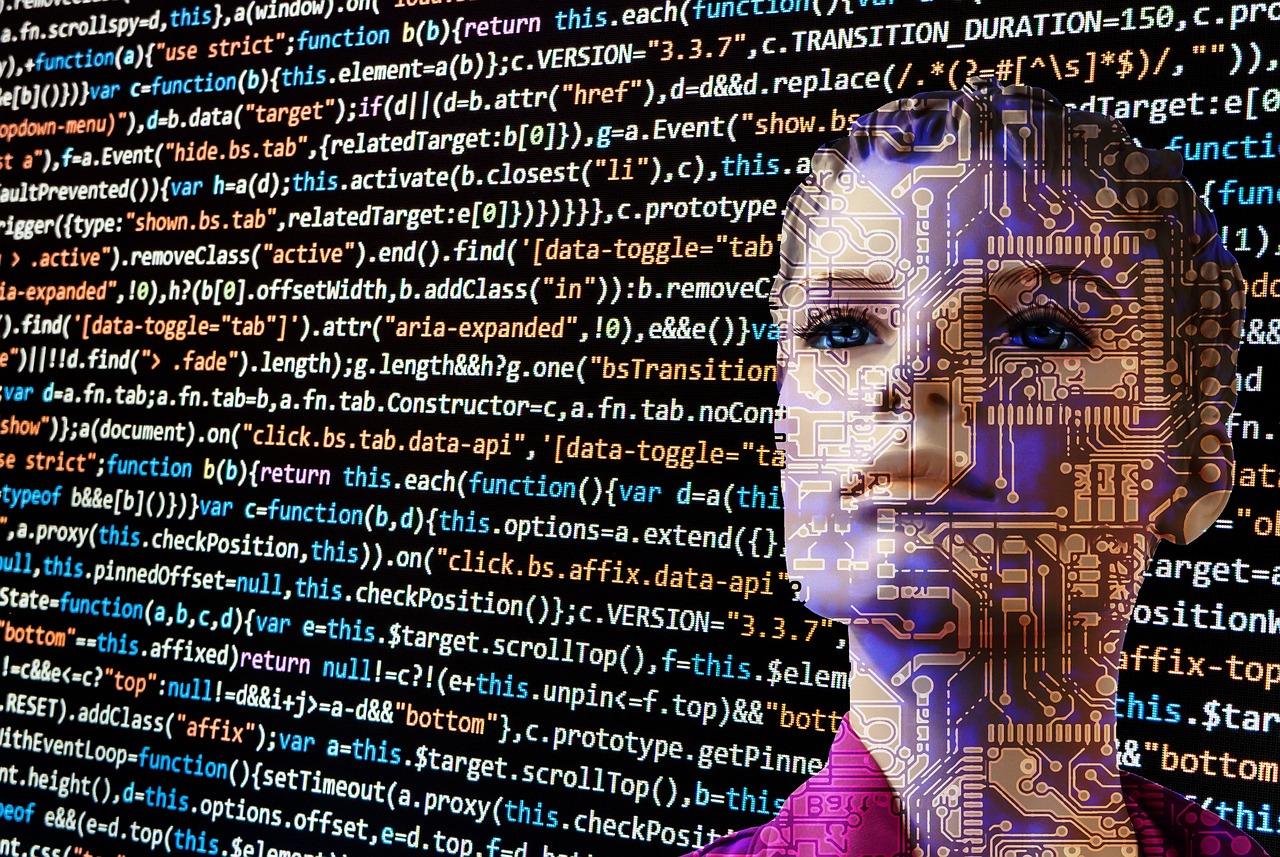
Artificial intelligence (AI) has been a hot topic in recent years, with rapid advancements and increased adoption across various industries. Despite its prevalence, many people still find AI to be a complex and mysterious subject. In this comprehensive guide, we aim to demystify AI and provide a better understanding of its history, applications, and future impact on society and the economy.
I. History of Artificial Intelligence
The concept of artificial intelligence dates back to ancient history, with myths and stories about artificially created beings endowed with intelligence or consciousness. However, the scientific pursuit of AI began in the mid-20th century.
- Early beginnings: The term “artificial intelligence” was coined in 1956 during the Dartmouth Conference, where researchers John McCarthy, Marvin Minsky, Nathaniel Rochester, and Claude Shannon proposed that “every aspect of learning or any other feature of intelligence can in principle be so precisely described that a machine can be made to simulate it.“
- Rule-based systems and expert systems: In the 1960s and 1970s, AI research focused on creating rule-based systems that relied on explicitly programmed knowledge and reasoning capabilities. Expert systems emerged as an important AI application, using rules to emulate the decision-making of human experts in specific domains.
- Connectionism and neural networks: In the 1980s, the idea of connectionism gained traction, inspired by the structure and function of the human brain. Artificial neural networks (ANNs) emerged as an alternative approach to AI, using interconnected nodes or neurons to process and learn from input data.
- Machine learning: The development of machine learning in the 1990s and early 2000s represented a major milestone for AI. Machine learning algorithms enabled AI systems to automatically learn and improve from experience, rather than relying on explicit programming.
- Deep learning: Since the 2010s, deep learning has emerged as a powerful subset of machine learning. Deep learning utilizes deep neural networks with multiple layers to automatically extract features from raw data, leading to significant advancements in AI capabilities.
II. Core Concepts and Techniques in AI
Understanding the key concepts and techniques in AI can provide a solid foundation for exploring its applications and potential impact.
- Machine learning: Machine learning is a method of AI that allows systems to automatically learn and improve from experience without being explicitly programmed. The learning process involves feeding data into the algorithms, which adjust their parameters to optimize performance.
- Supervised learning: In supervised learning, the AI system is trained on a dataset with labeled examples, learning to map input data to the corresponding output labels. This technique is commonly used for tasks such as classification and regression.
- Unsupervised learning: Unsupervised learning involves training AI systems on datasets without labeled output examples. The system learns to identify patterns, structures, or relationships within the data, typically used for clustering and dimensionality reduction.
- Reinforcement learning: In reinforcement learning, the AI system learns to make decisions by interacting with an environment, receiving feedback in the form of rewards or penalties. This technique is particularly suited for sequential decision-making tasks, such as game playing and robotics.
- Deep learning: Deep learning is a subset of machine learning that uses deep neural networks to automatically extract features and representations from raw data. This technique has proven particularly effective for image and speech recognition, natural language processing, and other complex tasks.
III. Applications of Artificial Intelligence
AI technologies have been applied across a wide range of industries and domains, revolutionizing the way we live and work.
- Healthcare: AI has been instrumental in improving diagnostics, personalized medicine, and drug discovery. Machine learning algorithms can analyze medical images and data to detect diseases, while AI-driven drug discovery accelerates the development of new treatments.
- Transportation: Self-driving cars and advanced driver assistance systems are prime examples of AI applications in transportation. AI technologies enable vehicles to perceive their environment, make decisions, and navigate autonomously, with the potential to significantly improve road safety and efficiency.
- Finance: AI has transformed the financial industry through fraud detection, algorithmic trading, and personalized financial planning. Machine learning algorithms can identify suspicious transactions, predict market trends, and create tailored investment strategies for individuals.
- Retail: AI-driven applications in retail include personalized recommendations, inventory management, and pricing optimization. By analyzing customer data and preferences, AI systems can suggest relevant products, streamline supply chain processes, and dynamically adjust prices based on demand.
- Manufacturing: AI technologies have revolutionized manufacturing through automation, predictive maintenance, and quality control. Robots powered by AI can perform complex tasks, while machine learning algorithms can predict equipment failures and optimize production processes.
- Entertainment: AI has made significant strides in content creation, recommendation systems, and game development. AI-generated music, art, and text are becoming more sophisticated, while recommendation algorithms help users discover new content tailored to their interests.
IV. Ethical Considerations and Future Impact
As AI continues to advance and permeate various aspects of our lives, it raises important ethical considerations and questions about its future impact on society and the economy.
- Job displacement: The increasing automation of tasks and industries may lead to job displacement and workforce restructuring. While new job opportunities may arise, it is crucial to consider the reskilling and upskilling of workers to adapt to the changing job market.
- Bias and fairness: AI systems can inadvertently perpetuate or amplify biases present in the training data or algorithms. Ensuring fairness and reducing bias in AI requires rigorous evaluation, transparency, and ongoing research.
- Privacy and security: The widespread use of AI and data-driven technologies raises concerns about privacy and data security. Striking a balance between leveraging data for AI applications and protecting user privacy is an ongoing challenge.
- Accountability and transparency: Determining responsibility for AI-driven decisions can be complex, particularly when systems are autonomous or rely on complex algorithms. Developing transparent AI systems and establishing clear lines of accountability is essential for building trust and addressing potential ethical concerns.
Conclusion
Artificial intelligence is a fascinating and rapidly evolving field, with transformative applications across numerous industries. By demystifying AI and understanding its core concepts, techniques, and implications, we can better appreciate its potential impact on society and the economy. As AI continues to advance, it is crucial to address the ethical considerations and challenges it presents, ensuring that its development benefits humanity as a whole.
Related Articles:
– How to Create High-Quality Digital Art Using AI Image Generators
– Virtual Thrills Await: Exploring Yas Island Top VR Experience
– The Remote Blueprint: Key Strategies for Establishing a Virtual Company